Machine Learning VS Automation: The Journey to AI
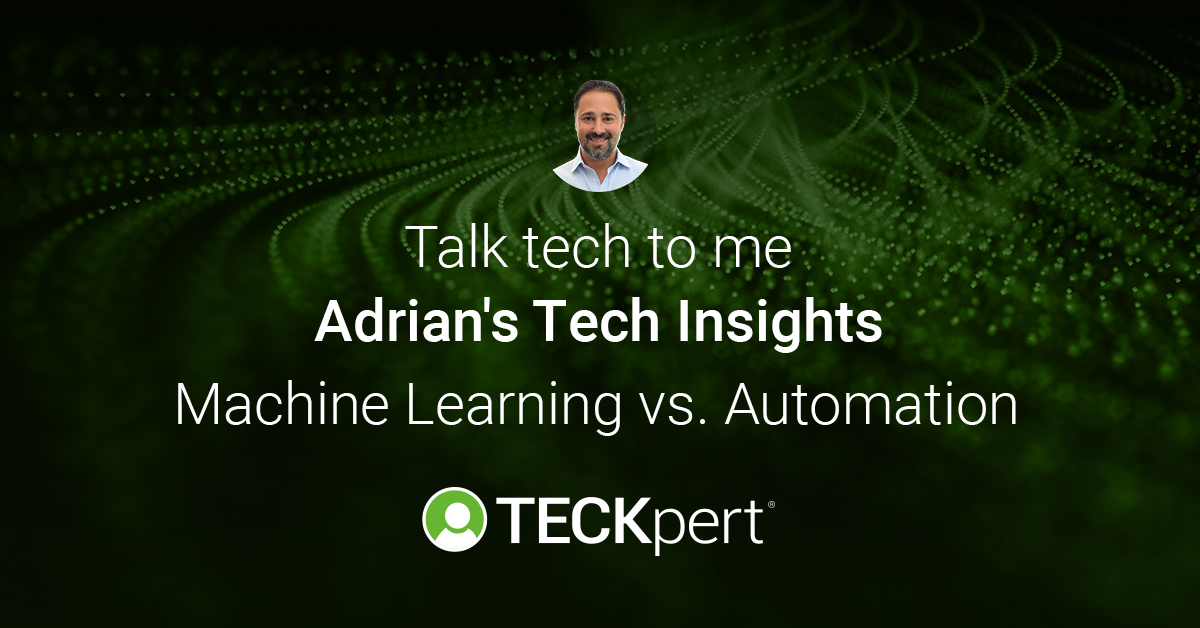
Machine learning and automation are two steps toward the same goal, Artificial Intelligence (AI). Separately, these approaches can be used to enhance processes and systems like apps, databases, and communication channels. Combined, they are two behaviors developing to support an ultimate predictive infrastructure.As with any journey, there are benefits and challenges along the way, so how might we use machine learning and automation to strengthen our practices now?
What is Automation?
The human body’s autonomic nervous system is largely subconscious. Breathing, pumping blood, and your base chemical reactions are all automated but these functions don’t continue past their set operations without permission from your conscious brain. Computer automation is the same. In fact, many digital functions have biological counterparts.These “subconscious” applications in your computer can be anything from automating email replies, to generating help desk tickets, or popups, to performing regular disk cleaning or virus scanning. The thing to remember is that automated processes exist only within their established functions, there is no capacity for independent dynamic action.
What is machine learning?
Machine learning is built on standardized analysis. While seemingly intelligent, interpreting data for prediction is a network of smaller, repetitive tasks geared toward identifying and flagging data based on preset parameters. This is just a fancy way of saying that machine learning isn’t teaching computers to think, but rather giving a system controlled choice - like a choose your own adventure book. The parameters are defined, but the computer makes a decision based on the information at hand and the previous data it has collected.By accumulating data through repetition, and analyzing it, machine learning is able to “predict” when to execute options; sending a reply, requesting a payment, notifying of a late transaction, etc. Where automated functions can run continuously without human interaction, machine learning is conducted primarily, (at least at the moment), for the purpose of analysis. It ‘’learns’’ by identifying key data points and flagging them for review and interpretation.
What Are We Trying to Achieve with Machine Learning and Automation?
The ultimate goal is Artificial Intelligence, but we’re not talking about world dominating robots just yet - nor are we talking about systems that will replace thousands of human jobs. For the moment, the successful combination of machine learning and automation is targeting more practical data applications; compiling resources, or interpreting incredibly vast amounts of data in a short time to support multiple industries, even medical and humanitarian efforts.AI is a comprehensive programming dream that layers data, automation, and machine learning with the added technologies of visual and language interpretation, and advanced processing that replicates a human’s freedom when reasoning. But let’s dial this way back.
In the Beginning
The journey to AI does not begin with automation or machine learning, it begins with digital data. The first step is converting your analog data into a digital format, like a spreadsheet or database. This data matrix then becomes the primary resource that fuels automated functions and informs machine learning systems. Data powers our modern world and is the magic ingredient that makes extensions like automation a possibility. Automation is the epitome of “work smarter not harder”, powering the instant data retrieval and automatic, auto-correct, auto-reply, landscape where we build our businesses and nurture our communities.
Capacity for the Future
One major ambition for machine learning is to apply it to widespread customer service and e-commerce. Machine Learning has the potential to influence metrics like ROI as it does not need to be programmed or watched in order to adapt and “evolve” to new data trends; this will influence everything from algorithms to analysis. A broad incorporation of machine learning in the commercial sector pushes us further down the road toward largely incorporating quantum computing into our systems - helping to increase performance and data processing to strengthen our resources and capacity for computing.
You Are Here.
While data manipulation can be automated, machine learning - which relies on a greater level of interpretation - is not as easily automated. This is where we are in the journey - our digital autonomic nervous system is a well-oiled machine but the machine learning aspects are mainly reserved for research, hefty development, and big data interpretation. We’ve got a little ways to go before our smart watches are instantly analyzing our diet and nutrition levels to tell us what we’re craving for dinner, but it’s inspiring to think about the opportunities that will emerge as we continue down the path toward data that is not only automated, but adaptive.A prevalent application for automation now, and a future landing pad for broadly applied machine learning, is dynamic data. Personalization is nearly mandatory for customer service and interaction when it comes to apps, welcome screens, security, and advertising, etc. Automated responses go hand-in-hand with a dynamic user experience, and machine learning will only enhance these features and, as a result, influence business strategies.Need help navigating the dynamic future of technology? Let our experienced tech experts map out your unique path forward for your organization. We have made it happen for many clients before and we look forward to our next interesting opportunity. Reach out today for an Assessment to discover what your business needs are for your next digital transformation – and how TECKpert can make it happen.